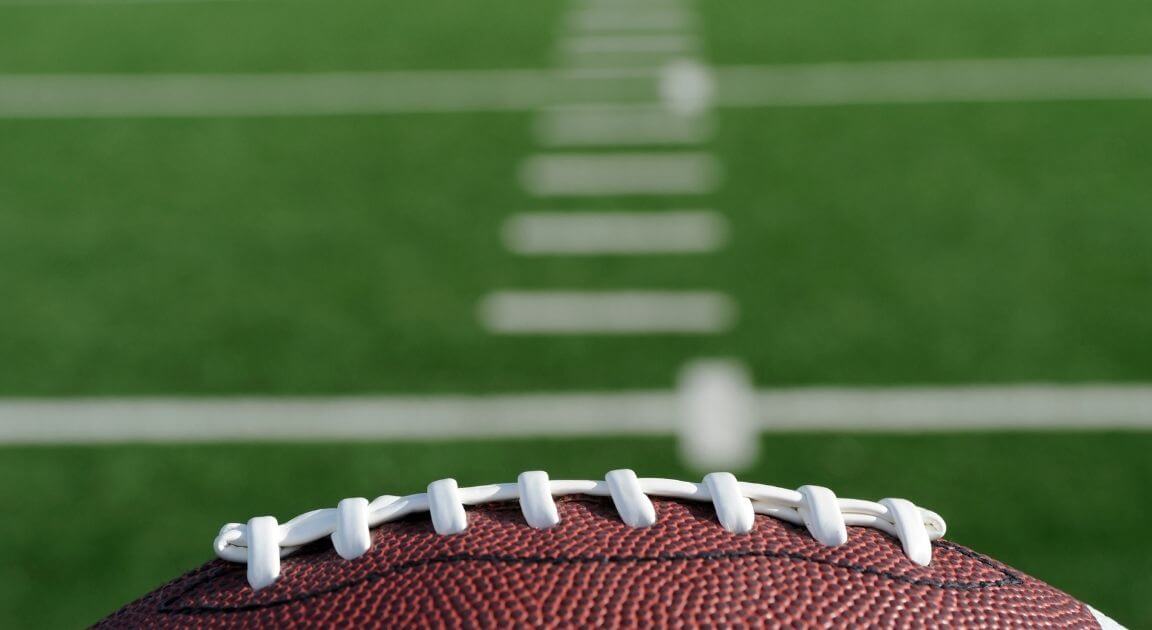
Coming off an exciting NFL playoff season, with all of the stats and information surrounding those games and broadcasts, it seems appropriate to look to the sports world for some examples of how to create a winning analytics formula for your business. Sports, like most industries, has seen a massive data boom in recent years. That data brings with it many possibilities, but also some important decisions.
What should you analyze, how do you analyze it, and how is it presented? Much of the attention often centers on the data collection and preparation process, and what the end result will look like. And rightfully so, as getting the data right typically is the most time-consuming and important component to ensure you have a solid foundation. And the end user experience can make or break adoption of new reports.
But how do you maximize the value of your reporting to truly gain an edge? To do so, choosing the right key performance indicators (KPIs), metrics, or analysis is paramount.
With our experience in the sports analytics industry, there are two concepts to consider when making decisions on KPIs and choosing the proper analyses: content and context.
Content – What is the metric actually measuring?
Context – Does the metric take into account the proper situational context?
Imagine a coach in the NFL evaluating a prospective quarterback. Total passing yardage may be a starting point, but yards per game or even yards per pass attempt will likely be more telling. Or, for that particular coach’s system, the percentage of completed passes may be more important for gauging future success. Contextually, the coach might want to consider the quality of opponents the player faced or even the quality of the player’s own teammates. Offensive proficiency in the NFL has also risen in recent years, so the benchmarks used to define high performance in the past may no longer be relevant.
While the problem you’re facing may be more simple or more complex than choosing the right quarterback, the same concepts likely apply to any business looking to leverage data to drive insight and make decisions. As you determine how best to measure your company’s production, progress, and success, here are three questions to consider:
1. What is the ultimate goal, and what available data will be most meaningful in showing progress toward that goal?
Back to our quarterback example, think about the LA Rams’ recent trade of Jared Goff for Matthew Stafford. Of course, the Rams’ ultimate goal is to win more games, and better players will help to accomplish that goal. Through the 2020 season, Goff’s Rams teams had won 59.5% of the games he started, compared to only a 44.3% winning percentage for Stafford’s Lions. But with several complicating factors (quality of teammates, coaching staffs, etc.), would the players’ past winning percentages truly be the best measure for judgment?
Using an advanced metric such as Quarterback Rating (QBR), which more accurately accounts for a quarterback’s contribution on the field, will do a better job of measuring each player’s impact on team performance. QBR (measured on a 0-100 scale where 50 represents a roughly average player) gives Stafford and his career average of 56.4 the clear edge over Goff (47.8).
Similarly, suppose you’re attempting to increase sales and would like to monitor your salespersons’ progress in doing so. Measuring overall sales will likely be the final benchmark for whether the goal was achieved. But perhaps tracking something like leads generated will provide more meaningful long-term insight into future sales and the true progress being made.
A salesperson who closed $5,000 in new sales over the previous month and generated 10 new leads might have done more to drive progress than another salesperson who generated $7,500 in new sales but only two new leads. You may want to incorporate product margins as well, as sales of higher-margin items will be more valuable to the business. Keep in mind that while traditional metrics are a good start, there may be less obvious data available that can help tell a more complete story.
2. Which “version” of your data will be most insightful?
Depending on the situation, you may need your data in its raw form, or it may require some adjustment. Without getting too deep into the weeds here, think about raw data as individual data points. That raw data could be adjusted by calculating a summed total, an average, or a percentage.
If a coaching staff is evaluating an opposing defense, should they look at the number of yards given up by that defense in each individual game? Or would yards per game, or even yards per play, average be more telling? Defense on third downs is usually of particular interest, as it measures a team’s ability to get the opposing offense off the field. However, knowing that the defense allowed 6.1 yards per play on third downs isn’t as useful without also knowing the distance to go for a first down in those situations. In this scenario, looking at each third down and indicating whether the offense gained a first down or not, then reporting that the defense allowed a third down conversion percentage of 73% may be more informative.
For your business, will it be more meaningful to look at your sales numbers with daily, weekly, or monthly totals? Or, if you’re trying to identify outliers that may be skewing a total or average, like an invoice amount of $10,000 when all other invoices are below $1,000, you may not want to aggregate at all. Maybe you’re grading your employees based on customer reviews. If the reviews are given on a scale of one to ten, is it more important to see that an employee has an average score of 8.7 or that 92% of that employee’s reviews have a score of eight or higher?
3. What is “good” and what is “bad”?
Even a well-planned and carefully designed metric may not mean much in a vacuum. Comparing benchmarks from previous periods or the current environmental standard can help add context.
An NFL quarterback passing for 25 touchdowns this past season may seem impressive without further information. But knowing that the league leaders have often been above 40 or even 50 touchdown passes in recent years adds context. When a major change occurs, whether the slower evolution of increased scoring over the past few decades or the more sudden league move from 16 regular season games to the 17 that were played this season, that benchmarking must adapt. In this example, comparing a quarterback’s season total touchdown passes to the league average for that same season (28.4 for quarterbacks that played at least 15 games in 2021) may be the best choice.
In the business world, comparing the current year to prior year sales can help add perspective. Be thoughtful when making these comparisons. Should the current month be compared to the prior month or the same month in the prior year? Does the metric in question need to be calculated in total or split by location, department, etc.? You may even want to include the comparison as part of the metric calculation. If your company filled 9,872 orders in 2021 and you’re comparing that to the 8,913 orders filled in 2020, reporting the year-over-year change of +959 might be more to the point than reporting the two numbers separately. If there are known industry or environmental changes that will affect comparisons across dates or locations, quantifying and accounting for those as best you can will improve accuracy as well.
While there may be certain industry standards depending on your company’s domain and reporting need, every business will have its unique aspects. Asking these questions as you navigate the analytical process will help keep your team on track and make your organization truly data-driven. If you have any questions, please contact us or learn more about our data analytics services.
Recent News & Insights
5 Ways to Maximize Your Chances of Employment
Finding a Lifelong Career
CMS Announces New Method II Billing Edits for Critical Access Hospitals
The Dollar and International Diversification + 4.30.25
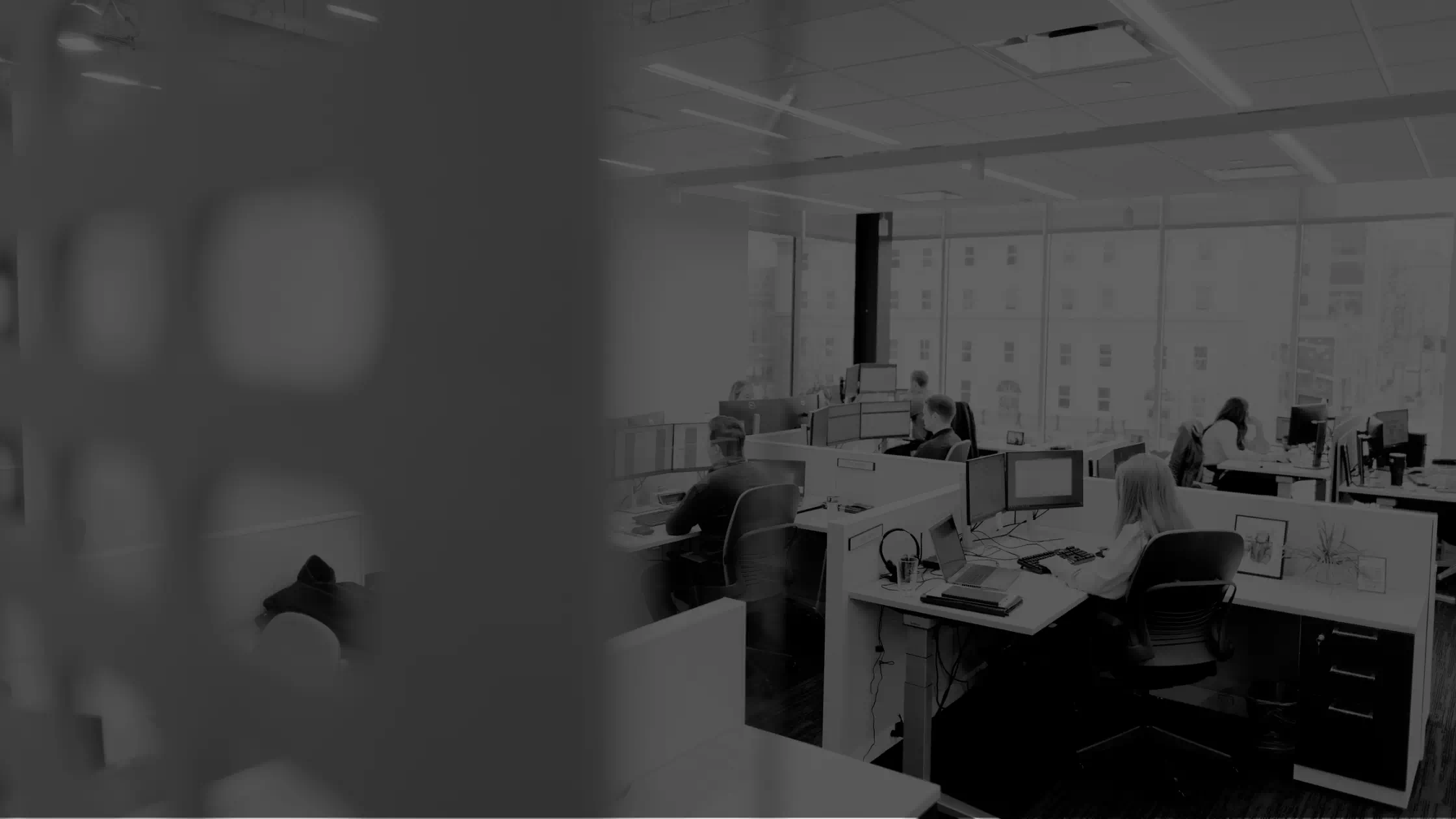
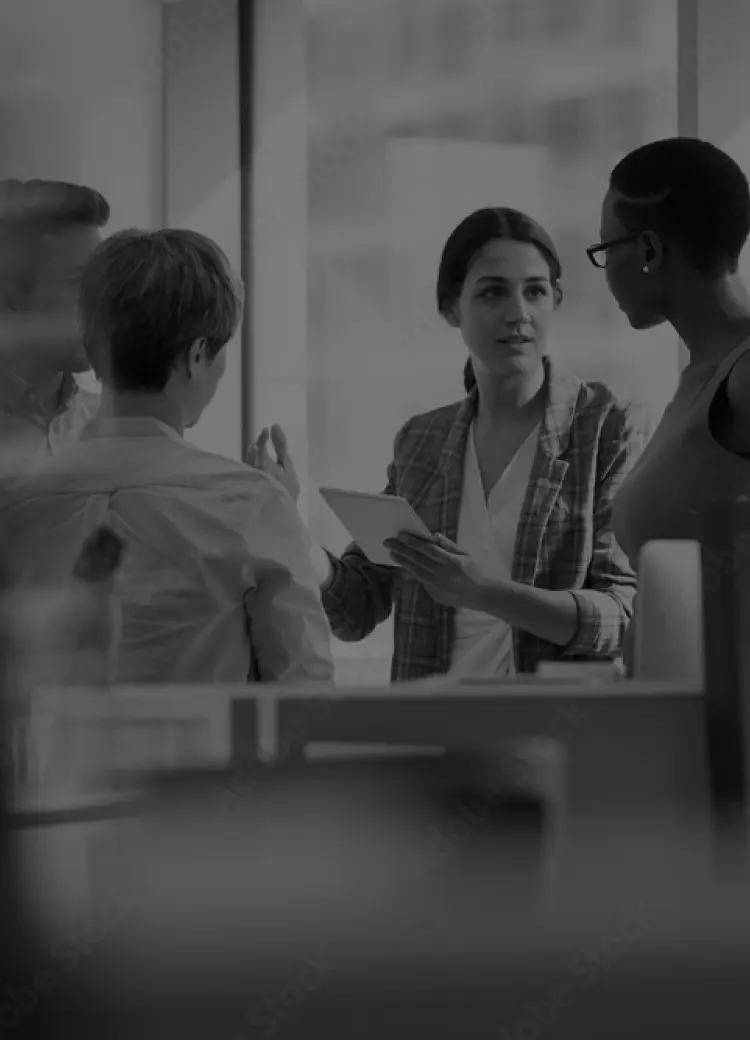